1 Introduction
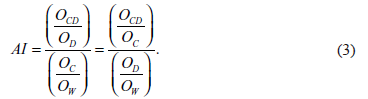
2 A Short Literature Study

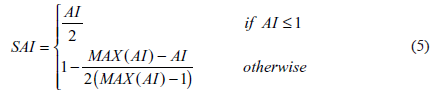
3 Reflections on the Meaning of the Activity Index
Table 1 Calculations related to Example A; the indicator F is introduced further on. |
Original situation | New situation: Country 2 publishes 17,000 articles less in other domains | |||||
---|---|---|---|---|---|---|
Country 1 | Country 2 | Country 1 | Country 2 | |||
OCD | 200 | 1,400 | 200 | 1,400 | ||
OD | 5,000 | 5,000 | 5,000 | 5,000 | ||
OC | 12,000 | 100,000 | 12,000 | 83,000 | ||
OW | 1,600,000 | 1,600,000 | 1,583,000 | 1,583,000 | ||
AI | 5.33 | 4.48 | 5.28 | 5.34 | ||
F | 0.0235 | 0.0267 | 0.0235 | 0.0318 |
Table 2 Data and calculations related to Example B. |
Basic | Increase in OCD | Decrease in OCD | |
---|---|---|---|
OCD | 190 | 200 | 180 |
OD | 200 | 210 | 190 |
OC | 200 | 210 | 190 |
OW | 400 | 410 | 390 |
AI | 1.9 | 1.859 | 1.945 |
F | 0.95 | 0.9524 | 0.9474 |
4 A New Proposal: F-measure for Research Priority

0 ≤ F ≤ 1, (7)


for research priority. Here and further on we exclude the trivial case that OCD =
OD = OC.
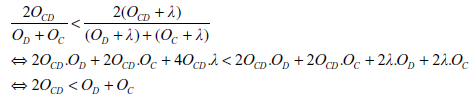
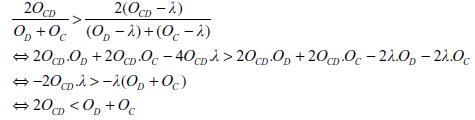
Theorem 2 (Relative independence)
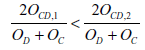



5 Further Mathematical Results
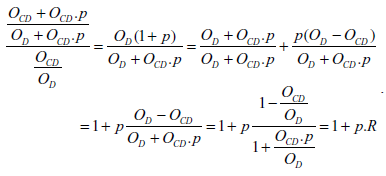
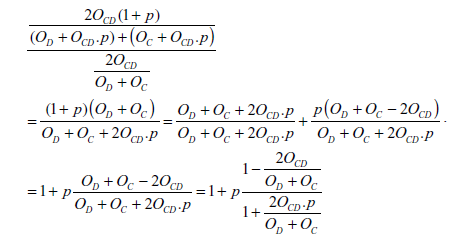

x = OCD/OD (the country’s share in the world’s publication in domain D) and y = OCD/OC (the domain’s share in the country’s publication output). As a function of x and y we have:

Figure 1. Graph of the function F(x,y); origin is nearest to the viewer. |
6 A Real-world Example
& Spruyt, 2012), came about as follows: In 2008 the Flemish government provided the legal framework for the construction of the Flemish Academic Bibliographic Database for the Social Sciences and Humanities (‘‘Vlaams Academisch Bibliografisch Bestand voor de Sociale en Humane Wetenschappen’’ or ‘‘VABB-SHW’’ in short). This database provided the Flemish government with a useful tool to fine-tune the distribution of research funding over universities in Flanders. As a consequence it became possible for researchers to analyze changing publication patterns in the larger Flemish peer reviewed literature (not just restricted to the WoS). Five publication types are included in the VABB-SHW:
Table 3 Flemish Humanities publications (2000-2009) in the VABB. |
Disciplines | Journal articles | Book chapters | Edited books | Monographs | Proceedings papers | Row totals | ||
---|---|---|---|---|---|---|---|---|
VABB-non-WoS | VABB-WoS | VABB | VABB | VABB | VABB-WoS | VABB-Non-WoS | ||
Archaeology | 176 | 133 | 40 | 6 | 11 | 12 | 18 | 396 |
Art History | 295 | 150 | 135 | 38 | 12 | 22 | 28 | 680 |
Communication Studies | 425 | 170 | 94 | 16 | 3 | 19 | 1 | 728 |
History | 773 | 193 | 233 | 52 | 28 | 0 | 19 | 1,298 |
Law | 4,018 | 144 | 320 | 89 | 55 | 11 | 20 | 4,657 |
Linguistics | 908 | 457 | 511 | 135 | 59 | 54 | 83 | 2,207 |
Literature | 631 | 143 | 376 | 87 | 36 | 0 | 31 | 1,304 |
Philosophy | 786 | 603 | 279 | 42 | 30 | 36 | 9 | 1,785 |
Theology | 610 | 85 | 410 | 85 | 53 | 1 | 4 | 1,248 |
Column totals | 8,622 | 2,078 | 2,398 | 550 | 287 | 155 | 213 | 14,303 |
Table 4 Values according to the AI-formula for the data shown in |
Disciplines | Journal articles | Book chapters | Edited books | Monographs | Proceedings papers | ||
---|---|---|---|---|---|---|---|
VABB-non-WoS | VABB-WoS | VABB | VABB | VABB | VABB-WoS | VABB-Non-WoS | |
Archaeology | 0.737 | 2.312 | 0.602 | 0.394 | 1.384 | 2.796 | 3.052 |
Art History | 0.720 | 1.518 | 1.184 | 1.453 | 0.879 | 2.985 | 2.765 |
Communication Studies | 0.968 | 1.607 | 0.770 | 0.572 | 0.205 | 2.408 | 0.092 |
History | 0.988 | 1.023 | 1.071 | 1.042 | 1.075 | 0.000 | 0.983 |
Law | 1.431 | 0.213 | 0.410 | 0.497 | 0.589 | 0.218 | 0.288 |
Linguistics | 0.682 | 1.425 | 1.381 | 1.591 | 1.332 | 2.258 | 2.525 |
Literature | 0.803 | 0.755 | 1.720 | 1.735 | 1.376 | 0.000 | 1.596 |
Philosophy | 0.730 | 2.325 | 0.932 | 0.612 | 0.838 | 1.861 | 0.339 |
Theology | 0.811 | 0.469 | 1.960 | 1.771 | 2.116 | 0.074 | 0.215 |
Table 5 Values according to the F-measure for the data shown in |
Disciplines | Journal articles | Book chapters | Edited books | Monographs | Proceedings papers | ||
---|---|---|---|---|---|---|---|
VABB-non-WoS | VABB-WoS | VABB | VABB | VABB | VABB-WoS | VABB-Non-WoS | |
Archaeology | 0.039 | 0.108 | 0.029 | 0.013 | 0.032 | 0.044 | 0.059 |
Art History | 0.063 | 0.109 | 0.088 | 0.062 | 0.025 | 0.053 | 0.063 |
Communication Studies | 0.091 | 0.121 | 0.060 | 0.025 | 0.006 | 0.043 | 0.002 |
History | 0.156 | 0.114 | 0.126 | 0.056 | 0.035 | 0.000 | 0.025 |
Law | 0.605 | 0.043 | 0.091 | 0.034 | 0.022 | 0.005 | 0.008 |
Linguistics | 0.168 | 0.213 | 0.222 | 0.098 | 0.047 | 0.046 | 0.069 |
Literature | 0.127 | 0.085 | 0.203 | 0.094 | 0.045 | 0.000 | 0.041 |
Philosophy | 0.151 | 0.312 | 0.133 | 0.036 | 0.029 | 0.037 | 0.009 |
Theology | 0.124 | 0.051 | 0.225 | 0.095 | 0.069 | 0.001 | 0.005 |
Table 6 Correlation values. |
Pearson | Spearman | |||||||
---|---|---|---|---|---|---|---|---|
PUB-AI | PUB-F | AI-F | PUB-AI | PUB-F | AI-F | |||
Journal articles VABB-non-WoS | 0.873 | 0.998 | 0.872 | 0.183 | 0.983 | 0.267 | ||
Journal articles VABB-WoS | 0.521 | 0.964 | 0.704 | 0.431 | 0.470 | 0.750 | ||
Book chapters VABB | 0.599 | 0.922 | 0.852 | 0.633 | 0.933 | 0.783 | ||
Edited books VABB | 0.621 | 0.789 | 0.965 | 0.500 | 0.683 | 0.933 | ||
Monographs VABB | 0.461 | 0.645 | 0.961 | 0.233 | 0.533 | 0.867 | ||
Proceedings papers VABB-WoS | 0.631 | 0.724 | 0.990 | 0.731 | 0.849 | 0.950 | ||
Proceedings papers VABB-Non-WoS | 0.595 | 0.734 | 0.979 | 0.633 | 0.800 | 0.933 |
Note. PUB stands for number of publications |
7 Discussion and Conclusion
Appendix


